CNPU: Mobile Deep RL Accelerator
본문
Overview
Recently, deep neural networks (DNNs) are actively used for object recognition, but also for action control, so that an autonomous system, such as the robot, can perform human-like behaviors and operations. Unlike recognition tasks, real-time operation is important in action control, and it is too slow to use remote learning on a server communicating through a network. New learning techniques, such as reinforcement learning (RL), are needed to determine and select the correct robot behavior locally. Fig. 7.4.1(a) shows an example of a robot agent that uses a pre-trained DNN without RL, and Fig. 7.4.1(b) depicts an autonomous robot agent that learns continuously in the environment using RL. The agent without RL falls down if the land slope changes, but the RL-based agent iteratively collects walking experiences and learns to walk even though the land slope changes.
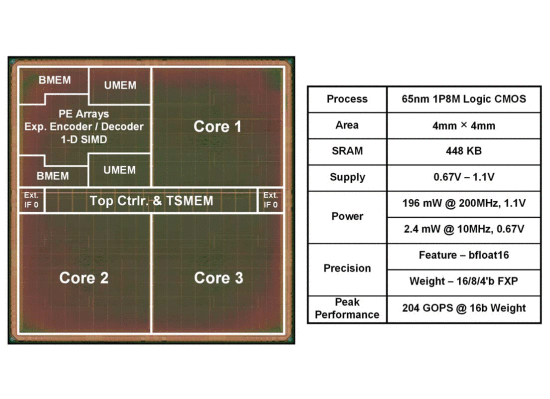
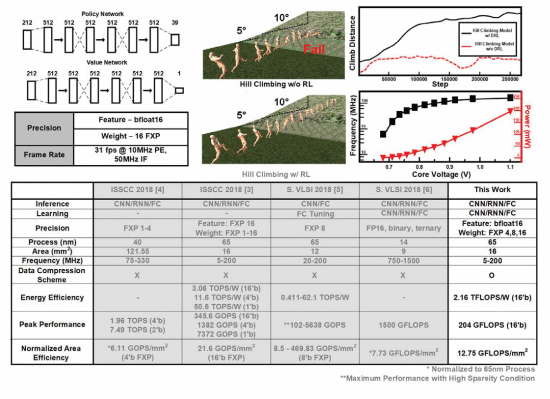

Features
- Energy Efficient Reconfigurable
- DRL Processor Experience Compressor for Reducing EMA
- Adaptive data reuse transposable PE array
Related Papers
- ISSCC 2019 [pdf]