CNN-Stereo-based 3D HGR SoC
본문
Overview
Recently, 3D hand-gesture recognition (HGR) has become an important feature in smart mobile devices, such as head-mounted displays (HMDs) or smartphones for AR/VR applications. A 3D HGR system in Fig. 13.4.1 enables users to interact with virtual 3D objects using depth sensing and hand tracking. However, a previous 3D HGR system, such as Hololens [1], utilized a power consuming time-of-flight (ToF) depth sensor (>2W) limiting 3D HGR operation to less than 3 hours. Even though stereo matching was used instead of ToF for depth sensing with low power consumption [2], it could not provide interaction with virtual 3D objects because depth information was used only for hand segmentation. The HGR-based UI system in smart mobile devices, such as HMDs, must be low power consumption (<;10mW), while maintaining real-time operation (<;33.3ms). A convolutional neural network (CNN) can be adopted to enhance the accuracy of the low-power stereo matching. The CNN-based HGR system comprises two 6-layer CNNs (stereo) without any pooling layers to preserve geometrical information and an iterative-closest-point/particle-swarm optimization-based (ICP-PSO) hand tracking to acquire 3D coordinates of a user's fingertips and palm from the hand depth. The CNN learns the skin color and texture to detect the hand accurately, comparable to ToF, in the low-power stereo matching system irrespective of variations in external conditions [3]. However, it requires >1000 more MAC operations than previous feature-based stereo depth sensing, which is difficult in real-time with a mobile CPU, and therefore, a dedicated low-power CNN-based stereo matching SoC is required.
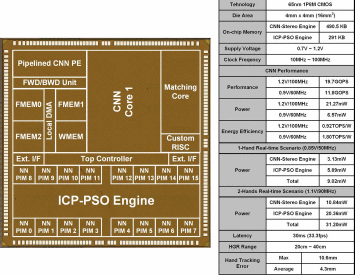
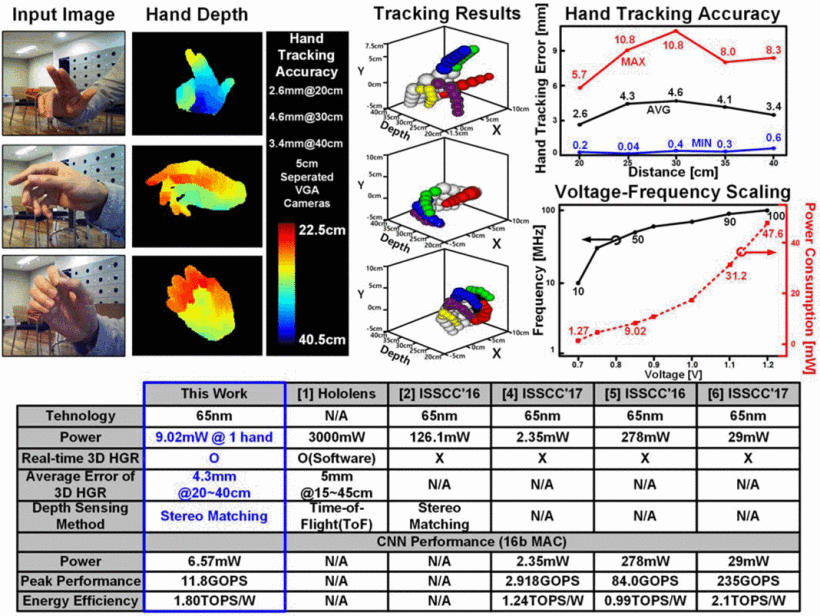
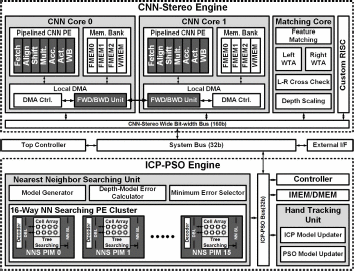
Features
- Pipelined CNN processing element (PE) with a shift MAC operation
- Triple ping-pong buffers with workload balancing
- Nearest-neighbor searching (NNS) processing-in-memory (PIM)
Related Papers
- ISSCC 2018 [pdf]
- 이전글CNPU: Mobile Deep RL Accelerator 19.02.08
- 다음글UNPU: Unified DNN ASIC for Mobile Deep Learning 19.02.08